Table Of Content
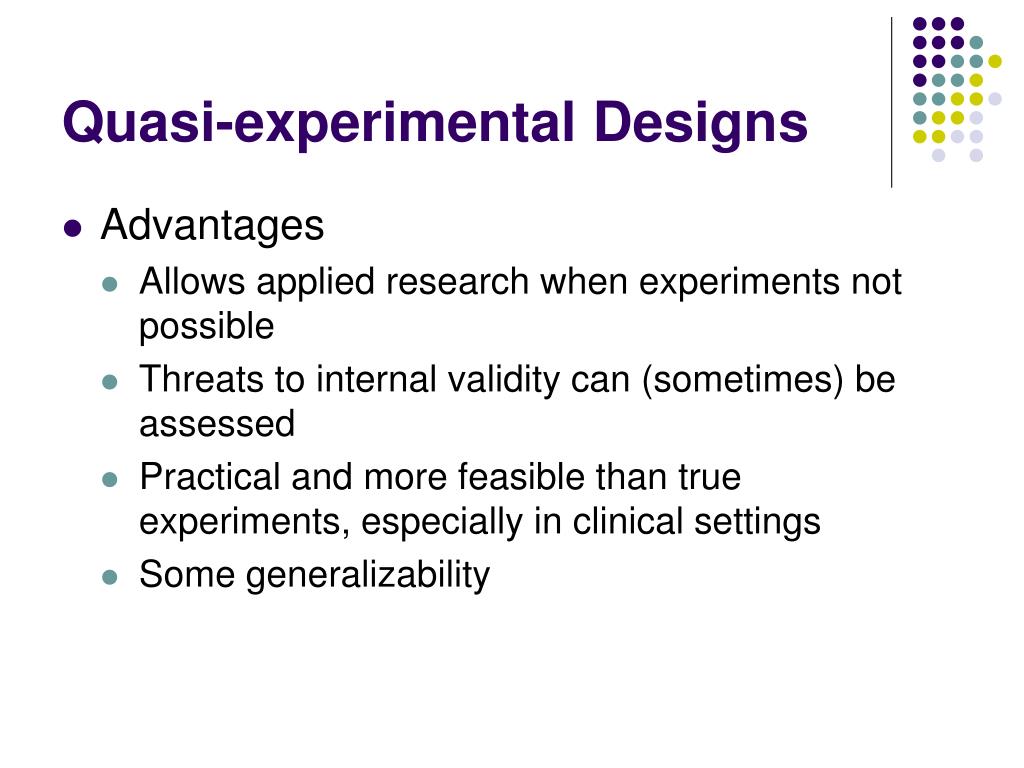
Implicit in this approach is the assumption that the greater the similarity between groups, the smaller the likelihood that confounding will threaten inferences of causality of effect for the intervention (33, 47). Thus, it is important to select this group or multiple groups with as much specificity as possible. One example of an implementation SMART is the Adaptive Implementation of Effective Program Trial (ADEPT; Kilbourne et al., 2014a). ADEPT was a clustered SMART (NeCamp et al., 2017) designed to inform an adaptive sequence of implementation strategies for implementing an evidence-based collaborative chronic care model, Life Goals (Kilbourne et al., 2014c; Kilbourne et al., 2012a), into community-based practices. Quasi-experiments are most likely to be conducted in field settings in which random assignment is difficult or impossible. They are often conducted to evaluate the effectiveness of a treatment—perhaps a type of psychotherapy or an educational intervention.
Interrupted Time Series
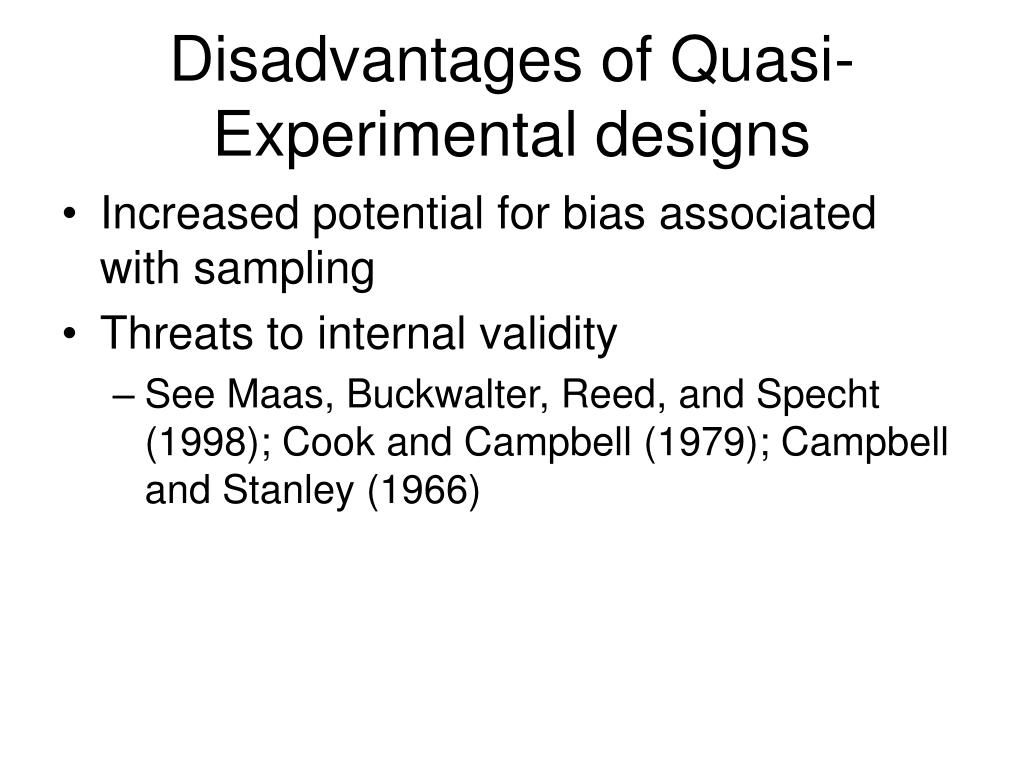
In fact, the students in professor l’s and 3’s courses may have scored high on the post-test without ever having been exposed to the treatment because they were biased at the pre-test. Some advantages of quasi-experimental studies are that, when compared to true experiments, they are less expensive and have higher external validity. However, because the researchers were not able to randomly assign children to one of the four parenting styles, the internal validity is called into question. True experiments, in which all the important factors that might affect the phenomena of interest are completely controlled, are the preferred design. Often, however, it is not possible or practical to control all the key factors, so it becomes necessary to implement a quasi-experimental research design.
Major Components of the Classic Experimental Design
The classic experiment is the foundation for other types of experimental and quasi-experimental designs. The unfortunate reality, however, is that the classic experiment, or other experimental designs, are few and far between in criminal justice.20 Recall that one of the major components of an experimental design is random assignment. Achieving random assignment is often a barrier to experimental research in criminal justice. Achieving random assignment might, for example, require the approval of the chief (or city council or both) of a major metropolitan police agency to allow researchers to randomly assign patrol officers to certain areas of a city and/or randomly assign police officer actions.
The Threat of Alternative Explanations
Another alternative explanation for a change in the dependent variable in a pretest-posttest design is regression to the mean. This refers to the statistical fact that an individual who scores extremely on a variable on one occasion will tend to score less extremely on the next occasion. For example, a bowler with a long-term average of 150 who suddenly bowls a 220 will almost certainly score lower in the next game. Regression to the mean can be a problem when participants are selected for further study because of their extreme scores. Imagine, for example, that only students who scored especially low on a test of fractions are given a special training program and then retested. Regression to the mean all but guarantees that their scores will be higher even if the training program has no effect.
Government Reforms and Economic Impact
A hypothetical quasi-experimental study was conducted in an economically developing country in a mid-sized city. For instance, it's impractical to trawl through large sample sizes of participants without using a particular attribute to guide your data collection. This type of research is often performed in cases where a control group cannot be created or random selection cannot be performed. Lessons from experiments of programs such as Scared Straight.” Crime and Delinquency, 46, 354–379.
Random assignment was utilized and this means that the groups have a higher probability of being equivalent on all factors that could impact the post-test. Thus, random assignment in this example helps to ensure the only differences between the groups are the treatment conditions. Without random assignment, there is a greater chance that one group of youth was somehow different, and this difference could impact the post-test. The pre-test in the example above allows the researcher to determine change in re-arrests from pretest to post-test. Thus, the researcher can determine if delinquent behavior, as measured by re-arrest, increased, decreased, or remained constant from pre- to post-test.
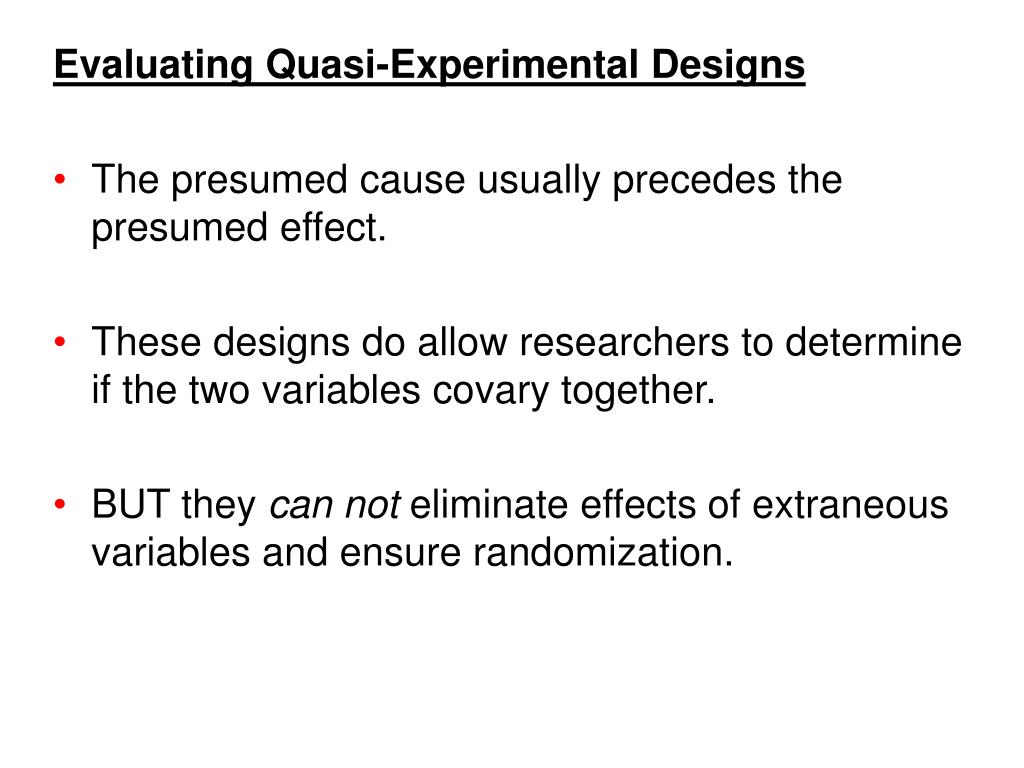
Stratification may be used to at least partially address confounding issues in cluster- randomized and more traditional trials alike, by ensuring that intervention and control groups are broadly similar on certain key variables. Furthermore, such allocation schemes typically require analytic models that account for this clustering and the resulting correlations among error structures (e.g., generalized estimating equations [GEE] or mixed-effects models; Schildcrout et al., 2018). This design involves the inclusion of a nonequivalent dependent variable (b) in addition to the primary dependent variable (a). Variables a and b should assess similar constructs; that is, the two measures should be affected by similar factors and confounding variables except for the effect of the intervention. Variable a is expected to change because of the intervention X, whereas variable b is not. Taking our example, variable a could be pharmacy costs and variable b could be the length of stay of patients.
Which quantitative data analysis tests should I use for ordinal data in quasi-experimental design? - ResearchGate
Which quantitative data analysis tests should I use for ordinal data in quasi-experimental design?.
Posted: Fri, 17 Apr 2015 07:00:00 GMT [source]
Random selection refers to selecting a smaller but representative sample from a larger population. For example, a researcher may randomly select a sample from a larger city population for the purposes of sending sample members a mail survey to determine their attitudes on crime. The goal of random selection in this example is to make sure the sample, although smaller in size than the population, accurately represents the larger population. There are certain situations where the use of a quasi-experimental design is more suited to the study.
Example of Quasi-Experimental Designs
What are some potential problems that could occur and how could those potential problems impact interpretation of results? How does the absence of an equivalent control group affect a researcher’s ability to determine the unique effects of the treatment on the outcomes being measured? Implementation science is focused on maximizing the adoption, appropriate use, and sustainability of effective clinical practices in real world clinical settings. Many implementation science questions can be feasibly answered by fully experimental designs, typically in the form of randomized controlled trials (RCTs). Implementation-focused RCTs, however, usually differ from traditional efficacy- or effectiveness-oriented RCTs on key parameters. Other implementation science questions are more suited to quasi-experimental designs, which are intended to estimate the effect of an intervention in the absence of randomization.
Interprofessional communication skills training to improve medical students' and nursing trainees' error communication ... - BMC Medical Education
Interprofessional communication skills training to improve medical students' and nursing trainees' error communication ....
Posted: Wed, 03 Jan 2024 08:00:00 GMT [source]
A counterbalanced design is a higher order study design than other studies in category A. In this design, all subjects receive the new PDA-based order entry system and the old computer terminal-based order entry system. The counterbalanced design is a within-participants design, where the order of the intervention is varied (e.g., one group is given software A followed by software B and another group is given software B followed by software A).
Quasi-experimental designs are useful in situations where randomized controlled trials are not feasible or ethical. They provide researchers with an alternative method to evaluate the effectiveness of interventions, policies, and programs in real-life settings. Quasi-experimental designs can also help inform policy and practice by providing valuable insights into the causal relationships between variables. One overarching drawback of this design is that it is especially vulnerable to threats to internal validity (Shadish, 2002), because pre-existing differences between the treatment and control group could erroneously be attributed to the intervention.
If particular types of individuals drop out or refuse to participate more often than individuals with other characteristics, this is called differential attrition. For example, suppose an experiment was conducted to assess the effects of a new reading curriculum. If the new curriculum was so tough that many of the slowest readers dropped out of school, the school with the new curriculum would experience an increase in the average reading scores.
This study design is not limited to two groups; in fact, the study results have greater validity if the intervention effect is replicated in different groups at multiple times. In the example of a pharmacy order-entry system, one could implement or intervene in the MICU and then at a later time, intervene in the SICU. This latter design is often very applicable to medical informatics where new technology and new software is often introduced or made available gradually. The reader should note that with all the studies in this category, the intervention is not randomized. Obtaining pretest measurements on both the intervention and control groups allows one to assess the initial comparability of the groups. The assumption is that if the intervention and the control groups are similar at the pretest, the smaller the likelihood there is of important confounding variables differing between the two groups.
In reviewing the results of several studies of treatments for depression, researchers Michael Posternak and Ivan Miller found that participants in waitlist control conditions improved an average of 10 to 15% before they received any treatment at all (Posternak & Miller, 2001)[2]. Thus one must generally be very cautious about inferring causality from pretest-posttest designs. Another alternative explanation for a change in the dependent variable in a pretest-posttest design is regression to the mean.
For example, prison inmates are not typically randomly assigned to different cellblocks by prison officers. Moreover, it is highly unlikely that a prison would have two open cellblocks waiting for a researcher to randomly assign incoming inmates to the prison for a tobacco ban study. Therefore, it is likely there would be differences among the groups in the quasi-experiment. The previous discussion is not to suggest that the nonequivalent group design cannot be useful in answering important research questions.